Abstract
The increased prominence of artificial intelligence/machine learning in youth’s lives has called for inclusion in computational literacies. Our focus on algorithm auditing—a practice which involves repeated examination of an algorithm’s output to make more transparent inner workings and possible impact—addresses key dimensions of artificial intelligence/machine learning literacies: (a) building on informal personal practices that youth engage with everyday technologies, (b) connecting with already existing computational thinking practices, and (c) integrating critical evaluation for algorithmic justice. In this presentation, we share findings from a study that positioned youth (ages 14-15) as peer-auditors. We conducted a two-week workshop in which youth designed machine-learning powered applications and later audited each other’s projects. We analyzed pre/post clinical interviews in which they were presented with auditing tasks. Our analyses show that in post interviews all youth identified algorithmic biases and inferred dataset and model design issues. Youth also discussed algorithmic justice and machine learning model improvements. Furthermore, youth reflected that auditing provided them new perspectives on model functionality and ideas to improve their own models. We discuss potential applications of algorithm auditing in K-12 education.
Bio Yasmin B. Kafai
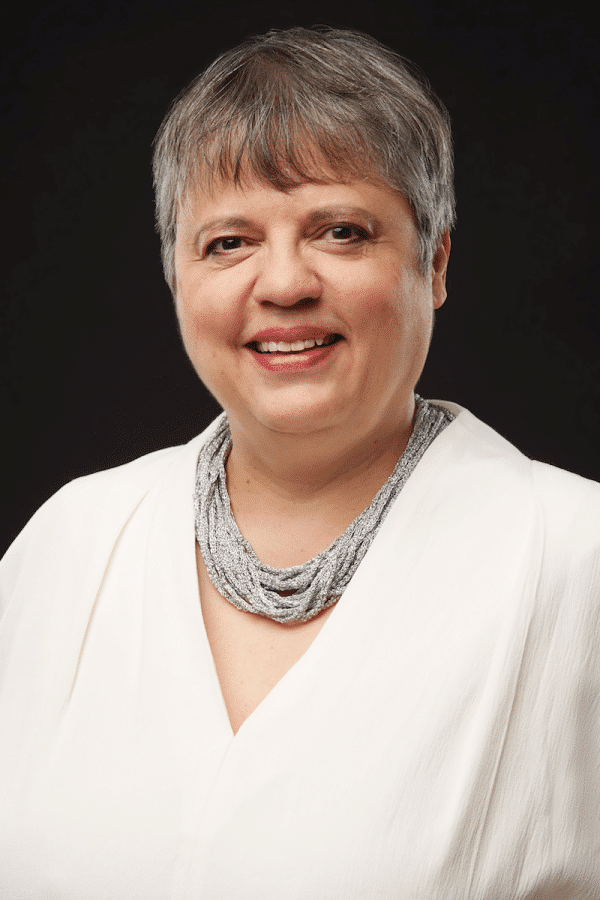
Luis Morales-Navarro
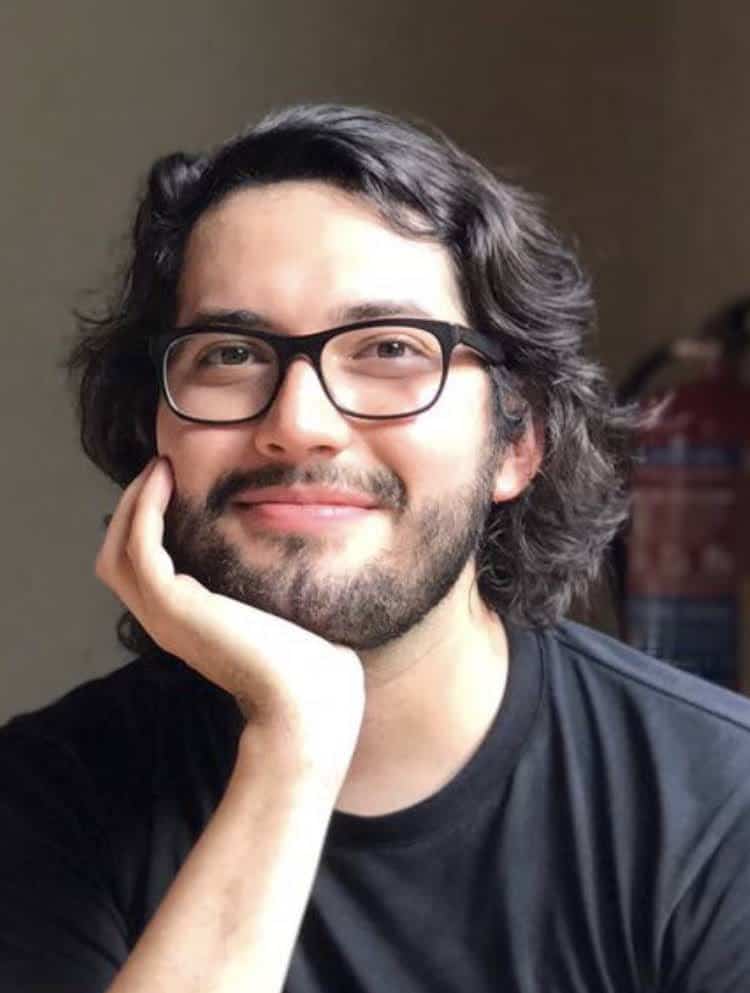
Luis Morales-Navarro is a doctoral student in the Learning Sciences and Technologies program at the University of Pennsylvania. His current research focuses on youth’s computational empowerment and studying novices’ understandings of machine learning systems. Previously he researched and designed tools and environments for learning computing at NYU’s Ability Lab, CMU’s Studio for Creative Inquiry, the Processing Foundation, Fundación Omar Dengo, and Apple.